Neuromorphic chips.
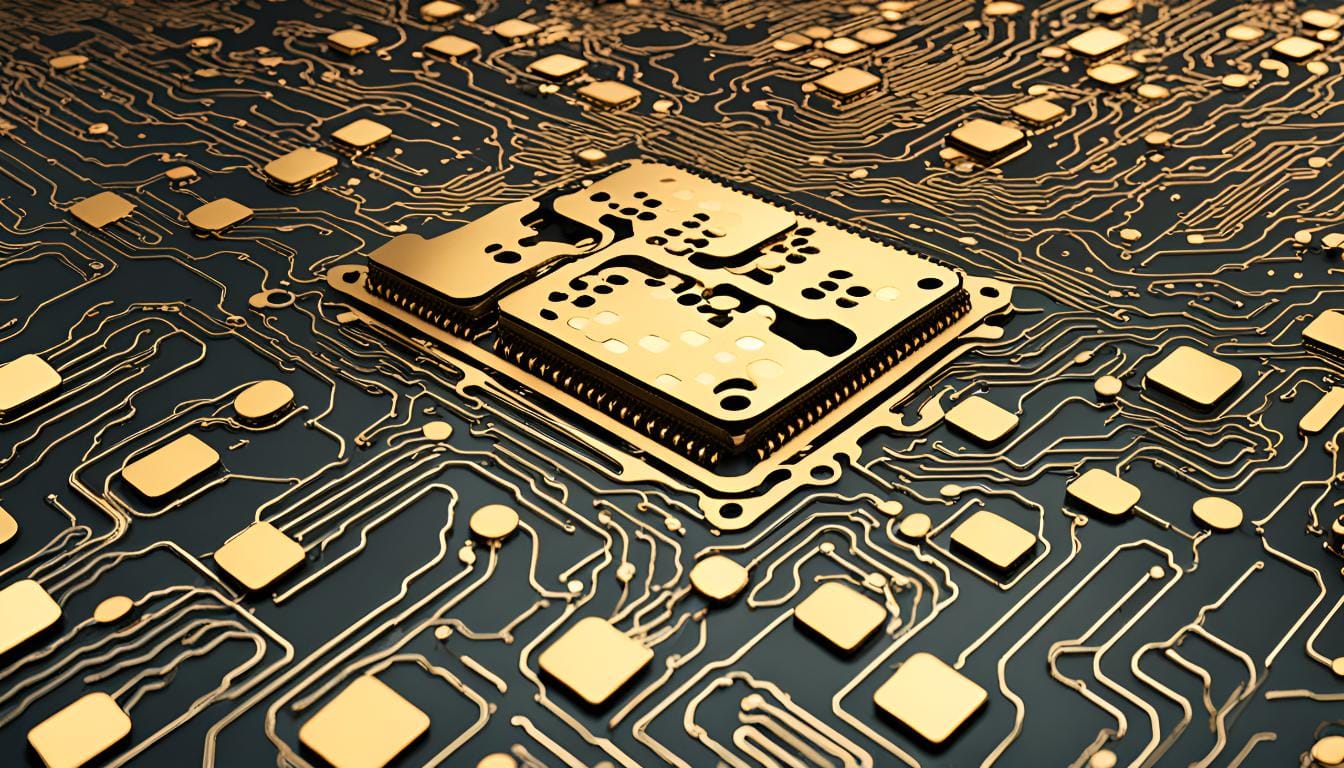
Today, we'll delve into the world of Neuromorphic Chips. These chips are inspired by the structure and function of the human brain, aiming to replicate its ability to learn, adapt, and process information efficiently.
History.
The concept of neuromorphic computing dates back to the 1940s when scientists like Alan Turing and John von Neumann began exploring the potential of building machines that could emulate human intelligence. However, the technological limitations of the time prevented significant advancements.
A resurgence of interest in neuromorphic computing occurred in the 1980s with the development of Artificial Neural Networks. These networks were inspired by the biological neural networks found in the brain. While they achieved some success in pattern recognition and machine learning, they were often computationally intensive and limited in their ability to replicate the brain's energy efficiency and real-time processing capabilities.
Neuromorphic Chips.
The primary goal of neuromorphic chips is to overcome the limitations of traditional computing architectures. By mimicking the brain's structure and function, these chips aim to:
►Improve energy efficiency: The human brain is incredibly energy-efficient compared to modern computers. Neuromorphic chips strive to replicate this efficiency, making them suitable for applications where power consumption is a critical factor.
►Enhance real-time processing: The brain can process information in parallel, allowing it to perform complex tasks in real-time. Neuromorphic chips aim to emulate this parallel processing capability, enabling them to handle tasks that are difficult or impossible for traditional computers.
►Enable more natural human-machine interactions: By operating in a more brain-like manner, neuromorphic chips could facilitate more intuitive and natural interactions between humans and machines.
AI, Neural Networks, and Neuromorphic Chips.
Despite being frequently used closely similar, these terms represent different ideas within the field of computing. A solid understanding of their contrasts is crucial for fully appreciating the subtleties of these technologies
►Artificial Intelligence (AI): AI is a broad field encompassing the development of intelligent agents that can reason, learn, and solve problems. AI can be implemented using various techniques, including neural networks and neuromorphic chips.
►Neural Networks: Neural networks are a subset of AI that are inspired by the structure and function of the human brain. They consist of interconnected nodes (neurons) that process information in parallel. While neural networks are often used in AI applications, they may not necessarily be neuromorphic.
►Neuromorphic Chips: Neuromorphic chips are specifically designed to mimic the brain's architecture and function at a hardware level. They are optimized for energy efficiency, real-time processing, and parallel computation.
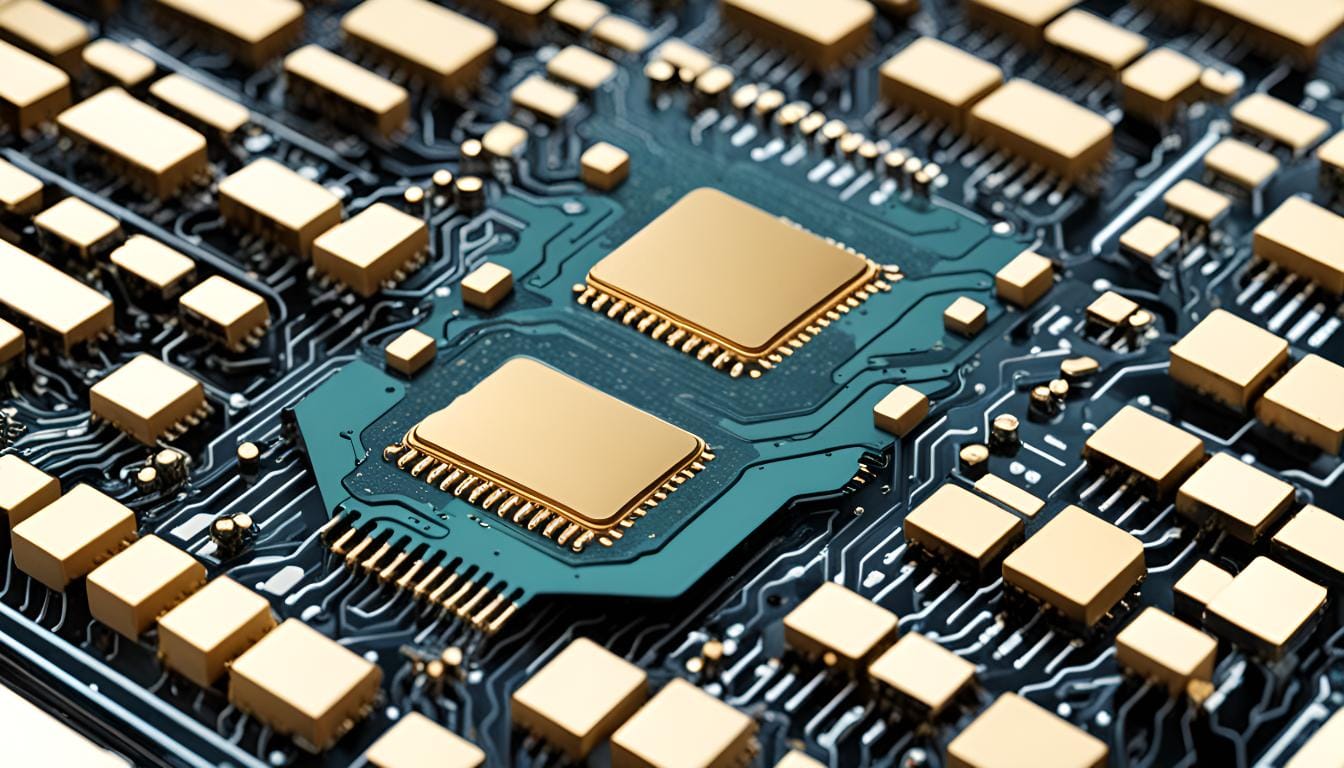
Future.
The future of neuromorphic chips is promising. As technology continues to advance, we can expect to see significant breakthroughs in this field. Some potential applications include:
►Edge computing: Neuromorphic chips could be used in edge devices (e.g., smartphones, drones, IoT devices) to enable real-time processing and decision-making without relying on cloud-based computing.
►Robotics: Neuromorphic chips could enable robots to learn from their environment and adapt to new situations more effectively.
►Healthcare: Neuromorphic chips could be used to develop more advanced prosthetics and brain-computer interfaces.
Conclusion.
Neuromorphic chips represent a fascinating and promising area of research. By leveraging the principles of the human brain, these chips have the potential to revolutionize computing and enable new and exciting applications.